人工智能算法模型在创伤患者下肢静脉血栓栓塞症诊断中的预测价值
Predictive value of artificial intelligence model in diagnosis of venous thromboembolism in lower extremities of trauma patients
摘要目的:探讨人工智能算法模型在创伤患者下肢静脉血栓栓塞症(VTE)诊断中的预测效能。方法:回顾性收集1992年12月至2017年11月中国人民解放军总医院信息系统数据库中骨折手术患者资料,共15 856例。按照患者有无血栓将其分组,并进行数据预处理和特征提取。选择随机森林(RF)、贝叶斯(Bayes)、决策树(DTC)及梯度提升树(GBDT)4种常用算法构建VTE风险预测模型,根据院内患血栓与否进行随机分层抽样,将原始数据按8∶2的比例分为训练集和测试集。通过比较上述方法中受试者工作特征曲线(ROC)下面积(AUC)、真阳性率(TPR)和精确度,评估不同模型在VTE临床诊断中的效能。根据研究特征在模型中的贡献程度进行重要特征排序,筛选VTE的重要预测特征。结果:RF、Bayes、DTC和GBDT模型AUC分别为0.89,0.86,0.68,0.71,TPR分别为0.29,0.44,0.38,0.66,精确度分别为0.97,0.94,0.95,0.76;其中RF模型AUC最大,精确度最高。对各VTE人工智能预测模型的重要特征分析表明,血栓病史可以作为不良结局的首要预测因素。RF模型重要临床特征排序为:血栓病史、依诺肝素钠注射液剂量、最后一次葡萄糖测定结果、术后首次葡萄糖测定结果等。结论:RF模型在创伤患者VTE风险预测中的精确度最高,能够为VTE预防策略制订提供参考依据。
更多相关知识
abstractsObjective:To explore the predictive value of artificial intelligence algorithm model for venous thromboembolism(VTE)in lower extremities of trauma patients.Methods:The data of 15,856 orthopedic inpatients were retrospectively collected from the information system database in Chinese PLA General Hospital from December 1992 to November 2017. The patients were divided according to whether they had thrombosis or not. Data pretreatment and feature extraction were carried out. Four artificial intelligence algorithms including Random Forest(RF),Bayes(Bayes),Decision Tree(DTC)and Gradient Boosting Tree(GBDT)were constructed to evaluate their clinical diagnostic efficacy in VTE. The original data were divided into training set and test set according to the ratio of 8∶2 by random stratified sampling method. By comparing the area under receiver operating characteristic curve(ROC)(AUC),true positive rate(TPR)and accuracy in the above methods,the efficiency of different models in clinical diagnosis of VTE was evaluated. According to the contribution degree of the features in the model,the important features were ranked to screen the independent risk factors of VTE.Results:For RF,Bayes,DTC and GBDT algorithm models,the AUC was 0.89,0.86,0.68,0.71,with the TPR for 0.29,0.44,0.38,0.66 and the accuracy for 0.97,0.94,0.95,0.76,respectively. The RF algorithm model had the highest accuracy and the largest AUC. Analysis of important features of artificial intelligence prediction models for VTE showed that the history of thrombosis was the primary predictor of adverse outcomes. The ranking of important clinical features represented by the RF model showed that the history of thrombosis,enoxaparin sodium injection dose,last glucose measurement and first glucose measurement after surgery were important predictive characteristics of VTE.Conclusions:The RF model has the highest accuracy in risk prediction of VTE in trauma patients,which can provide a reference for the formulation of VTE prevention strategies.
More相关知识
- 浏览0
- 被引12
- 下载0
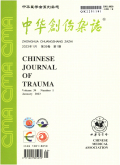
相似文献
- 中文期刊
- 外文期刊
- 学位论文
- 会议论文