融合空气数据的深度网络慢阻肺评估测试评分预测模型的构建及意义
Construction and significance of prediction model for chronic obstructive pulmonary disease assessment test based on fusion deep network fused with air data
摘要目的:构建融合空气数据的深度网络慢性阻塞性肺疾病(慢阻肺)评估测试(CAT)评分预测模型,并探讨其意义。方法:采用定组研究的方法,自2015年2月至2017年12月从北京大学第三医院、北京大学人民医院和北京积水潭医院呼吸科门诊入选稳定期的慢阻肺患者,采集患者住宅区附近的室外环境监测空气数据,计算患者每日空气污染物暴露量和天气数据,并连续记录患者每日的CAT评分。通过融合时序算法和神经网络建立模型对患者未来一周的CAT评分进行预测,并比较该模型与长短期记忆模型(LSTM)、添加全局注意力机制的LSTM模型(LSTM-attention)和自回归移动模型(ARIMA)的预测准确程度,并探讨预测模型的意义。结果:共纳入47例慢阻肺患者,平均随访时间为381.60 d,利用采集的空气数据和CAT评分构建长短期记忆模型-卷积神经网络-自回归(LSTM-CNN-AR)模型,其模型的均方根误差为0.85,平均绝对误差为0.71,较LSTM、LSTM-attention和ARIMA三者中最优模型平均预测准确度提升21.69%。结论:基于慢阻肺患者所处环境的空气数据,融合深度网络模型可更精准地预测慢阻肺患者的CAT评分。
更多相关知识
abstractsObjective:To construct a chronic obstructive pulmonary disease (COPD) assessment test (CAT) score prediction model based on a deep network fused with air data, and to explore its significance.Methods:From February 2015 to December 2017, the outdoor environmental monitoring air data near the residential area of the patients with COPD from the Respiratory Outpatient Clinics of Peking University Third Hospital, Peking University People′s Hospital and Beijing Jishuitan Hospital were collected and the daily air pollution exposure of patients was calculated. The daily CAT scores were recorded continuously. The CAT score of the patients in the next week was predicted by fusing the time series algorithm and neural network to establish a model, and the prediction accuracy of the model was compared with that of the long short-term memory model (LSTM), the LSTM-attention model and the autoregressive integrated moving average model (ARIMA).Results:A total of 47 patients with COPD were enrolled and followed up for an average of 381.60 days. The LSTM-convolutional neural networks (CNN)-autoregression (AR) model was constructed by using the collected air data and CAT score, and the root mean square error of the model was 0.85, and the mean absolute error was 0.71. Compared with LSTM, LSTM-attention and ARIMA, the average prediction accuracy was improved by 21.69%.Conclusion:Based on the air data in the environment of COPD patients, the fusion deep network model can predict the CAT score of COPD patients more accurately.
More相关知识
- 浏览0
- 被引0
- 下载0
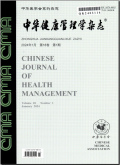
相似文献
- 中文期刊
- 外文期刊
- 学位论文
- 会议论文