基于CT检查影像组学在术前鉴别诊断胰腺浆液性囊腺瘤和黏液性囊腺瘤中的临床价值
Clinical value of radiomics based on CT examination in preoperative differential diagnosis of pancreatic serous cystadenoma and mucinous cystadenoma
摘要目的:探讨基于CT检查影像组学在术前鉴别诊断胰腺浆液性囊腺瘤(SCA)和黏液性囊腺瘤(MCA)中的临床价值。方法:采用回顾性病例对照研究方法。收集2012年1月至2019年12月浙江大学医学院附属第一医院收治的154例胰腺囊性肿瘤病人的临床病理和影像资料;男24例,女130例;年龄为(50±13)岁。154例病人中,99例为SCA,55例为MCA。154例病人术前均行胰腺CT平扫联合增强扫描检查。收集所有病人临床特征、影像特征、影像组学特征,构建临床特征模型、影像特征模型、影像组学模型和融合模型。绘制各个模型的受试者工作特征(ROC)曲线,并以曲线下面积(AUC)、准确度、灵敏度、特异度、阳性预测值和阴性预测值等指标评价构建模型。基于最优模型,构建列线图。观察指标:(1)临床特征模型建立及验证。(2)影像特征模型建立及验证。(3)影像组学模型建立及验证。(4)融合模型建立及验证。(5)融合模型的列线图。正态分布的计量资料以 x± s表示,组间比较采用Mann-Whitney U检验。计数资料以绝对数和(或)百分比表示,组间比较采用 χ2检验和Fisher确切概率法。 结果:(1)临床特征模型建立及验证:通过多元逻辑线性回归分析,筛选出年龄、临床症状、术前血清CA19-9 3个临床特征用于构建临床特征模型,多元逻辑线性回归结果以公式①表示:临床模型得分=0.635-0.007×年龄+0.054×临床症状+0.108×术前血清CA19-9。绘制临床特征模型测试集ROC曲线。临床特征模型训练集和测试集的AUC、准确度、灵敏度、特异度、阳性预测值、阴性预测值分别为0.611(95%可信区间为0.488~0.734, P<0.05)和0.771(95%可信区间为0.624~0.919, P<0.05)、56.6%和77.8%、66.7%和63.1%、56.3%和88.5%、41.5%和80.1%、78.4%和76.7%。(2)影像特征模型建立及验证:通过多元逻辑线性回归分析,筛选出肿瘤位置、肿瘤数目、肿瘤横断面最大径、肿瘤分叶、肿瘤多囊(≥6个)5个影像特征用于构建影像特征模型,多元逻辑线性回归结果以公式②表示:影像特征模型得分=-0.034+0.300×肿瘤位置+0.202×肿瘤数目+0.014×肿瘤横断面最大径-0.251×肿瘤分叶-0.170×肿瘤多囊(≥6个)。绘制影像特征模型测试集ROC曲线。影像特征模型训练集和测试集的AUC、准确度、灵敏度、特异度、阳性预测值、阴性预测值分别为0.862(95%可信区间为0.791~0.932, P<0.05)和0.853(95%可信区为0.713~0.994, P<0.05)、78.8%和88.9%、81.8%和89.4%、77.5%和88.5%、62.8%和85.0%、90.2%和92.0%。(3)影像组学模型建立及验证:本研究在154例胰腺囊性肿瘤病人中共提取4类1 067个影像组学特征,其中一阶直方图统计特征7个、纹理特征53个、小波特征848个、局部二值模式特征159个。在组内相关系数>0.9的条件下,共保留896个稳定性较好的影像组学特征用于构建模型。经过方差阈值与相关系数阈值筛选后,保留350个影像组学特征。为获得深层次的影像组学特征,在原特征基础上构建50个合成影像组学特征,总特征数为400个。采用五折递归特征消除后,最终筛选出22个影像组学特征,包括13个小波特征,7个合成影像组学特征和2个局部二值模式特征。采用支持向量机算法构建影像组学模型,模型惩罚系数“C”=35.938,模型参数“γ”=0.077,核函数为“径向基函数核”。绘制影像组学模型五折交叉验证的ROC曲线。影像组学模型的平均AUC、准确度、灵敏度、特异度、阳性预测值、阴性预测值分别为0.870( P<0.05)、83.1%、81.8%、83.8%、73.8%、89.2%。(4)融合模型建立及验证:选取影像特征的肿瘤位置、肿瘤分叶和影像组学得分构建融合模型。融合模型的多元逻辑线性回归结果以公式③表示:融合模型得分=-0.154+0.218×肿瘤位置-0.223×肿瘤分叶+0.621×影像组学得分。绘制融合模型测试集ROC曲线。融合模型训练集和测试集的AUC、准确度、灵敏度、特异度、阳性预测值、阴性预测值分别为0.893(95%可信区间为0.828~0.958, P<0.05)和0.966(95%可信区间为0.921~0.999, P<0.05)、83.7%和91.1%、81.8%和84.2%、84.5%和96.2%、71.1%和94.1%、90.9%和89.3%。(5)融合模型的列线图:绘制融合模型的列线图,模型约登指数为0.416。 结论:基于术前CT检查影像组学标签和影像特征的预测模型可鉴别诊断胰腺SCA和MCA。
更多相关知识
abstractsObjective:To investigate the clinical value of radiomics based on computed tomography (CT) examination in preoperative differential diagnosis of pancreatic serous cystadenoma (SCA) and mucinous cystadenoma (MCA).Methods:The retrospective case-control study was conducted. The clinicopathological and imaging data of 154 patients with pancreatic cystic neoplasms who were admitted to the First Affiliated Hospital, Zhejiang University School of Medicine from January 2012 to December 2019 were collected. There were 24 males and 130 females, aged (50±13)years. Of the 154 patients, 99 cases were diagnosed as SCA and 55 cases were diagnosed as MCA. All the 154 patients underwent plain and enhanced CT scan of pancreas before operation. The clinical characteristics, radiology features and radiomics features of all patients were collected to construct the clinical characteristics model, radiology model, radiomics model and fused model. The receiver operating characteristic (ROC) curve of each model was drawn, and those constructed models were evaluated by area under the curve (AUC), accuracy, sensitivity, specificity, positive predictive value and negative predictive value. Based on the optimal model, the nomogram was constructed. Observation indicators: (1) establishment and validation of clinical characteristics model; (2) establishment and validation of radiology model; (3) establishment and validation of radiomics model; (4) establishment and validation of fused model; (5) nomogram of fused model. Measurement data with normal distribution were represented as Mean± SD, and comparison between groups was analyzed using the Mann-Whitney U test. Count data were described as absolute numbers or percentages, and comparison between groups was analyzed using the chi-square test or Fisher exact probability. Results:(1) Establishment and validation of clinical characteristics model: 3 clinical characteristics, including age, symptoms and preoperative serum CA19-9, were selected using multinomial logistic linear regression analysis to construct the clinical characteristics model. Result of the multinomial logistic linear regression analysis was expressed by formula ①: clinical characteristics model score=0.635-0.007×age+0.054×clinical symptoms+0.108×preoperative serum CA19-9. The ROC curve for the test dataset of clinical characteristics model was drawn. The AUC, accuracy, sensitivity, specificity, positive predictive value and negative predictive value of clinical characteristics model were 0.611(95% confidence interval as 0.488?0.734, P<0.05), 56.6%, 66.7%, 56.3%, 41.5%, 78.4% for the training dataset and 0.771(95% confidence interval as 0.624?0.919, P<0.05), 77.8%, 63.1%, 88.5%, 80.1%, 76.7% for the test dataset, respectively. (2) Establishment and validation of radiology model: 5 radiology characteristics, including tumor location, the number of tumors, tumor diameter of cross section, lobulated tumor and polycystic tumor (more than 6), were selected using multinomial logistic linear regression analysis to construct the radiology model. Result of the multinomial logistic linear regression analysis was expressed by formula ②: radiology model score=?0.034+0.300×tumor location+0.202×the number of tumors+0.014×tumor diameter of cross section?0.251×lobulated tumor?0.170×polycystic tumor (more than 6). The ROC curve for the test dataset of radiology model was drawn. The AUC, accuracy, sensitivity, specificity, positive predictive value and negative predictive value of radiology model were 0.862(95% confidence interval as 0.791?0.932, P<0.05), 78.8%, 81.8%, 77.5%, 62.8%, 90.2% for the training dataset and 0.853(95% confidence interval as 0.713?0.994), P<0.05), 88.9%, 89.4%, 88.5%, 85.0%, 92.0% for the test dataset, respectively. (3) Establishment and validation of radiomics model: 4 categories of a total 1 067 radiomics features were extracted from 154 patients with pancreatic cystic neoplasms, including 7 first-order histogram features, 53 texture features, 848 wavelet features and 159 local binary pattern features. A total of 896 stable radiomics features were retained to construct the model, based on the condition of intraclass correlation coefficient >0.9. After selected by variance threshold and correlation coefficient threshold, 350 radiomics features were retained. Fifty synthetic radiomics features were constructed based on the original features in order to obtain potential radiomics features, and the total number of radiomics features was 400. After analyzed by the five-fold recursive feature elimination, 22 radiomics features were screened out, including 13 wavelet features, 7 synthetic radiomics features and 2 local binary pattern features. The support vector machine algorithm was used to construct the radiomics model. The penalty coefficient 'C' and parameter 'γ' of the radiomics model were 35.938 and 0.077, respectively. The kernel function of the radiomics model was 'radial basis function kernel'. The ROC curve of radiomics model using 5-fold cross validation was drawn. The average AUC, accuracy, sensitivity, specificity, positive predictive value and negative predictive value of the radiomics model were 0.870 ( P<0.05), 83.1%, 81.8%, 83.8%, 73.8% and 89.2%, respectively. (4) Establishment and validation of fused model: the fused model was constructed after selecting the tumor location and lobulated tumor of radiology characteristics and radiomics score. Result of the multinomial logistic linear regression analysis was expressed by formula ③: fused model socre=?0.154+0.218×tumor location?0.223×lobulated tumor+0.621×radiomics score. The ROC curve for the test dataset of fused model was drawn. The AUC, accuracy, sensitivity, specificity, positive predictive value and negative predictive value of fused model were 0.893(95% confidence interval as 0.828?0.958, P<0.05), 83.7%, 81.8%, 84.5%, 71.1%, 90.9% for the training dataset and 0.966(95% confidence interval as 0.921?0.999, P<0.05), 91.1%, 84.2%, 96.2%, 94.1%, 89.3% for the test dataset, respectively. (5) Nomogram of fused model: the nomogram of fused model was illustrated with the Youden index of 0.416. Conclusion:The prediction model based on the radiomics signature and radiological features extracted from preoperative CT examination can make the differential diagnosis of pancreatic SCA from MCA.
More相关知识
- 浏览0
- 被引6
- 下载0
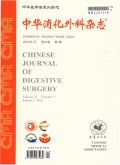
相似文献
- 中文期刊
- 外文期刊
- 学位论文
- 会议论文