摘要Induction of common knowledge or regularities from large-scale clinical data is a vital task for Chinese medicine(CM).In this paper,we propose a data mining method,called the Symptom-Herb-Diagnosis topic(SHDT)model,to automatically extract the common relationships among symptoms,herb combinations and diagnoses from large-scale CM clinical data.The SHDT model is one of the multi-relational extensions of the latent topic model,which can acquire topic structure from discrete corpora(such as document collection)by capturing the semantic relations among words.We applied the SHDT model to discover the common CM diagnosis and treatment knowledge for type 2 diabetes mellitus(T2DM) using 3 238 inpatient cases.We obtained meaningful diagnosis and treatment topics(clusters) from the data,which clinically indicated some important medical groups corresponding to comorbidity diseases(e.g.,heart disease and diabetic kidney diseases in T2DM inpatients).The results show that manifestation sub-categories actuallty exist in T2DM patients that need specific,individualised CM therapies.Furthermore,the results demonstrate that this method is helpful for generating CM clinical guidelines for T2DM based on structured collected clinical data.
更多相关知识
- 浏览22
- 被引15
- 下载0
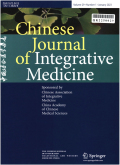
相似文献
- 中文期刊
- 外文期刊
- 学位论文
- 会议论文