利用深度学习方法预测鼻咽癌患者放疗疗程中的解剖图像
Prediction of anatomical images during radiotherapy of nasopharyngeal carcinoma with deep learning method
摘要目的:利用深度学习预测鼻咽癌患者疗程中的解剖图像,用于及时发现鼻咽癌患者放疗疗程中可能发生的解剖结构变化。方法:收集2020年1月1日至2022年12月31日于中国医学科学院肿瘤医院就诊的230例T 3-T 4期鼻咽癌患者的图像资料,包括计划CT(pCT)和各分次的锥形线束CT(CBCT)图像。使用3D Unet神经网络预测放疗第 k+1周的解剖图像,输入包括pCT、第1-3天的CBCT和第2- k周的各1次CBCT。训练了4个模型分别用于预测第3-6周的解剖图像。在预测图像和真实图像上勾画出鼻咽原发肿瘤(GTV nx)和左右腮腺,通过评估预测图像和真实图像上勾画结构的一致性来评估模型性能。 结果:本研究提出的方法能较准确预测整个放疗疗程中的解剖图像。预测与真实图像上感兴趣轮廓的一致性较高,GTV nx、左腮腺、右腮腺的戴斯相似性系数平均值分别为0.96、0.90、0.92,平均豪斯多夫距离分别为3.28、4.18、3.86 mm,平均最小距离分别为0.37、0.70、0.60 mm。 结论:利用深度学习方法预测鼻咽癌患者疗程中解剖图像的方法准确可行,有助于提前预测和准备治疗策略,实现个性化治疗。
更多相关知识
abstractsObjective:To develop a deep learning method to predict the anatomical images of nasopharyngeal carcinoma patients during the treatment course, which could detect the anatomical variation for specific patients in advance.Methods:Imaging data including planning CT (pCT) and cone-beam CT (CBCT) for each fraction of 230 patients with T 3-T 4 staging nasopharyngeal carcinoma who treated in Cancer Hospital Chinese Academy of Medical Sciences from January 1, 2020 to December 31, 2022 were collected. The anatomical images of week k+1 were predicted using a 3D Unet model with inputs of pCT, CBCT on days 1-3, and CBCT of weeks 2- k. In this experiment, we trained four models to predict anatomical images of weeks 3-6, respectively. The nasopharynx gross tumor volume (GTV nx) and bilateral parotid glands were delineated on the predicted and real images (ground truth). The performance of models was evaluated by the consistence of the delineation between the predicted and ground truth images. Results:The proposed method could predict the anatomical images over the radiotherapy course. The contours of interest in the predicted image were consistent with those in the real image, with Dice similarity coefficient of 0.96, 0.90, 0.92, mean Hausdorff distance of 3.28, 4.18 and 3.86 mm, and mean distance to agreement of 0.37, 0.70, and 0.60 mm, for GTV nx, left parotid, and right parotid, respectively. Conclusion:This deep learning method is an accurate and feasible tool for predicting the patient's anatomical images, which contributes to predicting and preparing treatment strategy in advance and achieving individualized treatment.
More相关知识
- 浏览0
- 被引0
- 下载0
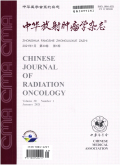
相似文献
- 中文期刊
- 外文期刊
- 学位论文
- 会议论文